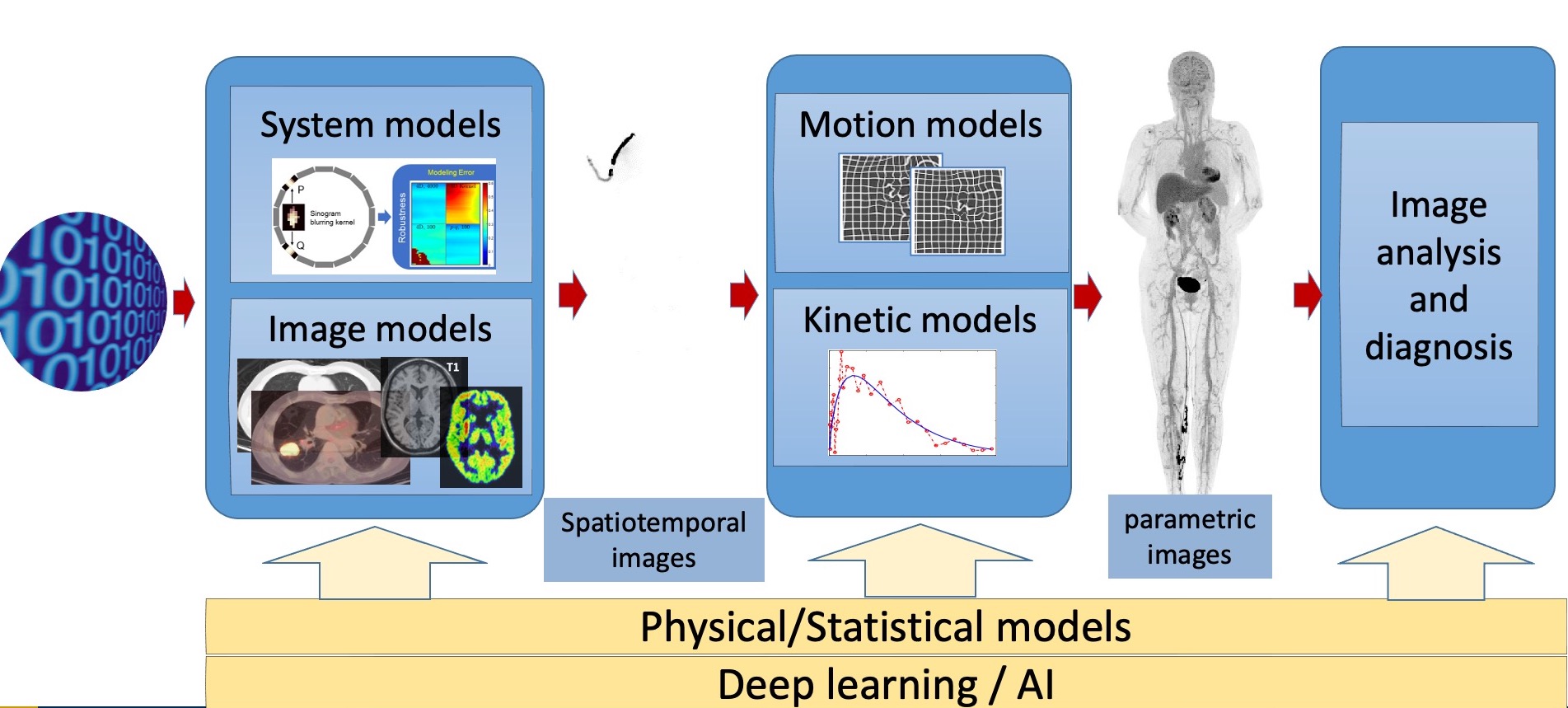
- Positronium lifetime imaging
- NeuroExploer
- Deep learning in PET and CT imaging
- Ultra-high-temporal-resolution dynamic PET
- Parametric imaging using dynamic PET
- High-resolution Imaging Reconstruction
- Dynamic PET Data Analysis
Positronium lifetime carries information about the tissue microenvironment where positrons are emitted. The lifetime information can be used to understand the tissue microenvironment in vivo which could facilitate the study of disease mechanism and selection of proper treatments. However, such information has not been captured because of two technical challenges. One challenge is the low sensitivity in detecting triple coincidence events. This problem has been mitigated by the recent developments of PET scanners with long (1-2 m) axial field of view. The other challenge is the lack of proper image reconstruction methods to produce high-resolution lifetime images. We are developing new image reconstruction methods to address the second challenge. Please see our TMI paper for more details.
Related news: Bangyan won the 1st place Best Student Paper Award at IEEE MIC for the SIMPLE method that we developed.
The NeuroEXPLORER (NX) project is a collaboration between Yale, UC Davis, and United Imaging Healthcare America is to develop a next-generation dedicated brain PET system, funded by a $10.2M BRAIN Initiative Grant from NIH. Motivated by experience with human brain HRRT studies (Yale) and total-body uEXPLORER studies (UC Davis), the NX design focused on ultra-high sensitivity and resolution, with continuous head motion correction. The NX has a cylindrical design with a system diameter of 52.4 cm and long axial field-of-view (FOV) of 49.5 cm. The detector micro-block consists of a 4x2 array of 1.56x3.07x20 mm LYSO crystals decoded by two 3-mm SiPMs including depth-of-interaction (DOI) measurement using an optical bridge for light-sharing between axial crystal pairs. To obtain high-resolution images, we have developed a new image reconstruction method with multiple image-domain point spread functions (PSFs).
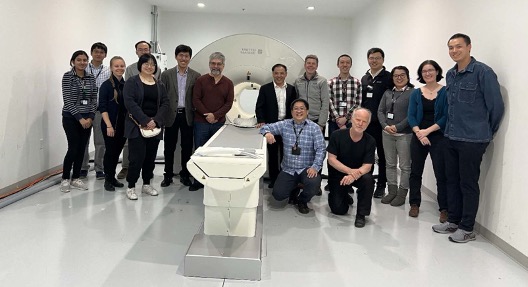
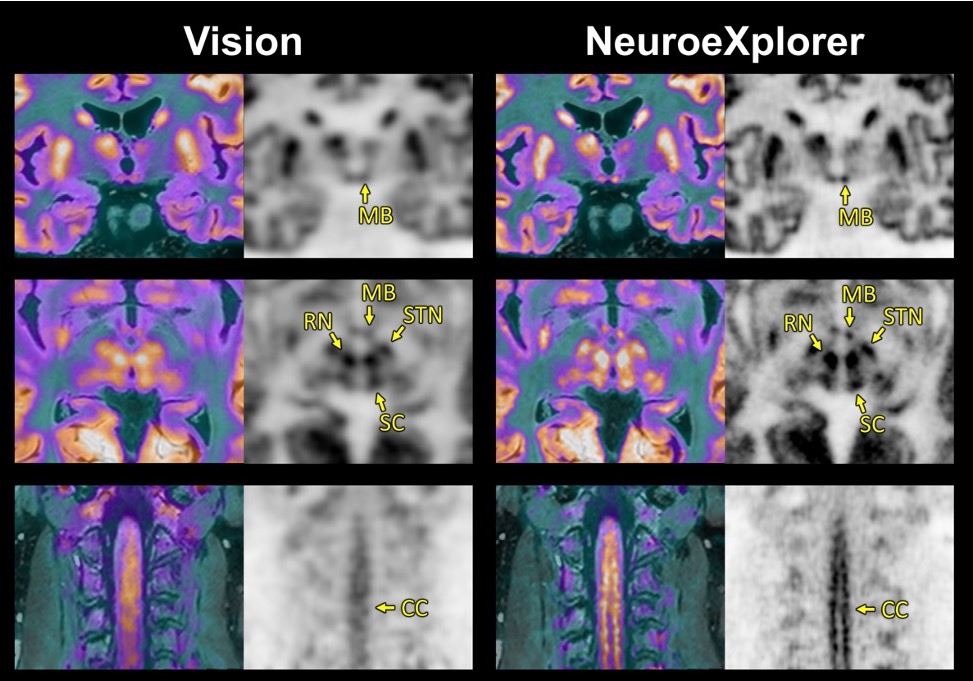
Related news: Tiantian won the 1st place PIDSC Young Investigator Award at the SNMMI annual meeting in Chicago for her multiple PSFs work.
Deep learning in PET and CT imaging
Deep learning has many applications in medical imaging from image acquisition, image reconstruction, image analysis, feature extraction, to clinical predictions. A few examples of our published work are listed here.
- Deep Learning Based Joint PET Image Reconstruction and Motion Estimation
- Anatomically aided PET image reconstruction using deep neural networks
- Motion correction of respiratory-gated PET images using deep learning based image registration framework
- AI-Based Automated Lipomatous Tumor Segmentation in MR Images: Ensemble Solution to Heterogeneous Data
- Head and neck synthetic CT generated from ultra‐low‐dose cone‐beam CT following Image Gently Protocol using deep neural network
- Half2Half: deep neural network based CT image denoising without independent reference data
Ultra-high-temporal-resolution dynamic PET
PET is capable of monitoring the spatial-temporal distribution of injected radiotracer inside a human body in real time. Using the EXPLORER scanner, we have demonstrated dynamic PET at 100-ms temporal resolution following a bolus injection of FDG in a human volunteer.
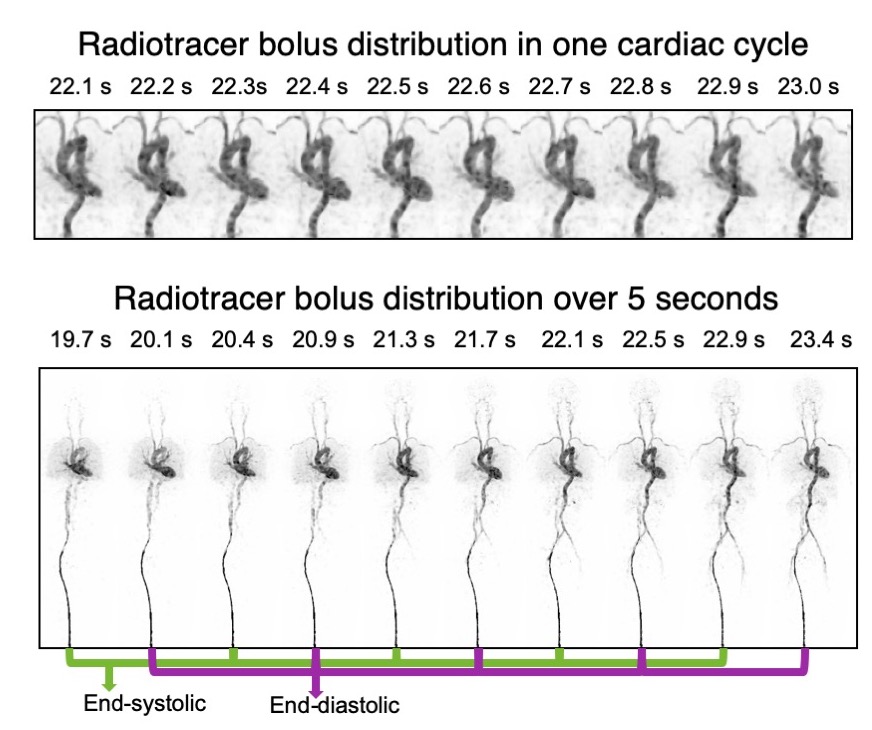
See our PNAS paper: subsecond total-body dynamic PET
Media coverages:
- MedicalXpress: Look what’s inside: Full-body movies from EXPLORER scanner
- News Medical: EXPLORER scanner captures real-time videos of blood flow and heart function
- Physics World: Ultrasensitive PET enables sub-second total-body imaging
Parametric imaging using dynamic PET
The total-body coverage and ultra-high sensitivity of the EXPLORER scanner provide opportunities for more accurate tracer kinetic analysis in studies of physiology, biochemistry, and pharmacology. We have developed quantitative parametric image reconstruction methods for kinetic analysis and used them to analyze the first human dynamic total-body PET study.
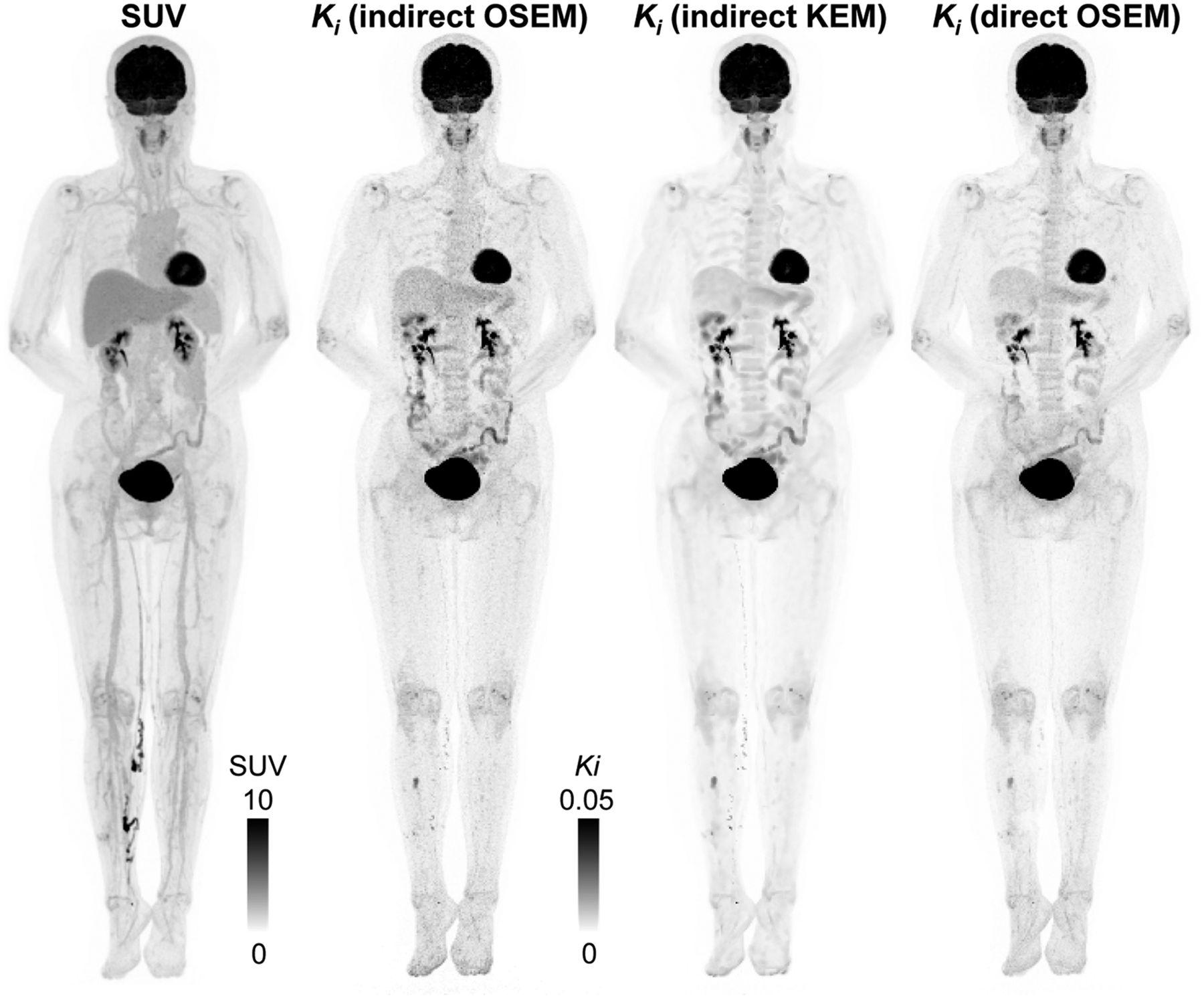
See our JNM paper for details: total-body dynamic PET and parametric imaging
High-Resolution Image Reconstruction
The resolution of current animal PET systems is on the order of millimeter. Images with higher resolution will greatly improve our capability of studying human diseases using mouse models. For example, sub-millimeter resolution would be required in order to detect metastases or study brain function in mice. While tremendous advance has been made in detector designs, sophisticated data processing techniques are necessary to realize its full potential. We are working on several fronts to push the resolution beyond the current limit, including super-resolution with continuous bed motion, estimation of detector blurring matrix from point source data, objective assessment of image quality for detection and quantification, and error correction in reconstruction algorithms. We have also proposed an adaptive zoom-in PET design that incorporates a high-resolution detector into an existing PET scanner to obtain high-resolution images of a targeted region with high sensitivity and developed a method to find the optimal position of the high-resolution detector. Shown below are some example images. Further readings: Tohme and Qi (2009), Lin and Qi (2010), Zhou and Qi (2009). |
||||||||||||||||||
![]() |
||||||||||||||||||
Reconstructed images of a simulated phantom using the microPET II scanner (left) and the zoom-in PET (right). Substantial improvement in spatial resolution is achieved by incorporating a high-resolution detector into the microPET II scanner. | ||||||||||||||||||
![]() |
||||||||||||||||||
High-resolution PET image obtained using our method (right) compared with the image obtained using the manufacture’s software (left).
|